Neural Object Detector im Vergleich zu Notate ML Nutzung und Statistiken
Neural Object Detector was designed to be used by both developers and people who are enthusiastic about Machine Learning, Computer Vision, and Object Detection / Image Classification using the combination of both. Neural Object Detector, by default is bundled with YOLOv3 model, which is a neural network for fast object detection that detects 80 different classes of objects. In addition to that, the app allows the users to import any custom machine learning model designed for object detection or image classification, with a single tap, the downloaded model can be imported via the Files app import window which is available within the app by simply pressing the plus icon on the models view so users do not have to leave the app. The app, based on the model selected, draws a rounded rectangle over the detected objects, the annotated image can be rendered and saved to photos or shared if the user chooses to share it directly from the app. To meet every users needs a handful of settings for computer vision algorithm and camera resolution can be changed. Users have the option to enable CPU only mode, which helps test their models under that specific condition.
In addition to Object Detection, Neural also supports Image Classification, by default, Neural is bundled with Resnet50 Image Classification Model.
Core ML* models which are a type of "Pipeline" in the format of *.mlmodel is supported by this app for Object Detection. No other model format is supported as of now.
Core ML Supported Tools, Services, and Converters:
• Turi Create* - https://github.com/apple/turicreate
• IBM Watson Services* - https://developer.apple.com/ibm/
• Core ML Tools* - https://pypi.org/project/coremltools/
• Apache MXNet* - https://github.com/apache/incubator-mxnet/tree/master/tools/coreml
• TensorFlow* - https://github.com/tf-coreml/tf-coreml
• ONNX* - https://github.com/onnx/onnx-coreml
* Turi Create, IBM Watson Services, Core ML, Apache MXNet, TensorFlow, ONNX might be registered trademarks of their respected owners / proprietors. Neural Object Detector nor the developer is not affiliated with any of the above services or companies.
YOLOv3 Model bundled with the is app is free, open source model. More info: https://github.com/pjreddie/darknet
Resnet50 Model bundled with this app for Image Classification is free, and open source model.
More info: https://github.com/fchollet/deep-learning-models/blob/master/LICENSE
Visit https://hariharanm.com/neural/acknowledgements/ for Acknowledgments.
The machine learning aspects are all proceed on device so nothing leaves the device. No cloud services are involved. No private analytics services. If, a user decides to contact app support a handful of data will be complied into a log file and attached to the mail composer, these data include app version, app configuration, device model, battery info, device software version, CPU utilised my the app. If the user decided not share, they can simply delete it and continue with the composing the support mail. No data is that is individually identifiable is collected.
https://hariharanm.com/
- Apple App Store
- Bezahlt
- Entwickler-Tools
Rang speichern
- -
Preparing input image datasets is a critical and challenging task in any Machine Learning project. Notate ML brings the power and usability of Apple's mobile devices to accelerate this task and deliver higher quality training data to your object detection model.
FEATURES
・Create a dataset, type, scan, or speak your labels into it
・Snap new pictures, or import old ones from your photo library
・Crop images, draw bounding boxes, tag and label objects of interest
・Export images and annotations for training with YOLO, Apple Create ML, or Google Auto ML
CREATING A DATASET
・Create a new data set and give it a unique name
・Add labels in one of several ways:
・One at a time, using the keyboard
・Copy and paste a comma-separated list from another document
・Scan from a physical text source
・Use the device voice feature to speak them in
・Labels can be edited or deleted by swiping left on each row
・Only unused labels can be deleted
IMPORTING IMAGES
・Tap into the dataset and import photos (up to 50 at a time) from your Photo Library
・This app can only access photos that you select using Apple's photo picker feature
CAPTURING IMAGES FROM THE CAMERA
・While browsing the images in a dataset, tap the "Capture" button to snap new images with the camera
・The app will require your permission to access the camera
・If you choose to decline, you can always grant access at a later date using "Settings"
ANNOTATING THE IMAGE
・Select an image from the dataset image browser to annotate it
ZOOMING and CROPPING
・Pinch with two fingers to zoom in or out of the image
・Drag with two fingers to move (pan) the image about
・Optionally crop the image to the visible square dimensions
・Cropping can only be done when the image has no bounding boxes.
・Double tap on the image to reset zoom and recenter the image
・Tap the "Reset" button in the bottom panel to restore image and annotations to the original state before editing
・The cropped image dimensions and visible area dimensions in pixels are displayed above the image at all times
TAGGING
・Drag with one finger or stylus to draw bounding boxes around objects of interest
・Long press on a box to select an existing annotation
・Use the picker to assign a label to the selected bounding box
・Or tap the red Delete button to remove the selected box
・Tap anywhere outside the selected box to unselect it
・Tap the "Reset" button to restore image and annotations to the original state before editing
・Tap the "Done" button to complete annotating the image
EXPORTING THE DATASET
・While browsing dataset images, tap the "Export" button, to select export options
・Datasets can be exported in one of three "schemas" for use with different object detector training frameworks
・The YOLO schema generates a set of files organized for use with the YOLO framework
・The Create ML schema generates files to use as input to Apple's Create ML application
・The Auto ML schema generates files to use as input to Google's Auto ML service
・ After reviewing the export options, tap the "Export" button on the Export options screen to generate data files for training
・ Choose one of the sharing options (e.g. Airdrop, Files) enabled by your device to export the data files
DELETING
・Delete an image by swiping left in the dataset image browser
・Delete an entire dataset by swiping left in the dataset browser
・If a dataset contains images, you will be prompted for confirmation
Some tips
・Crop the images before tagging them
・Use a stylus for more accurate bounding boxes
・Maintain different datasets for training and validation
・Limit datasets to under 1000 images each, for ease of use
・Export and delete unused datasets to conserve storage
- Apple App Store
- Kostenlos
- Entwickler-Tools
Rang speichern
- -
Neural Object Detectorvs. Notate ML Ranking-Vergleich
Vergleichen Sie Neural Object Detector den Ranking-Trend der letzten 28 Tage mit Notate ML
Neural Object Detector#73
Rang
Neural Object Detector im Vergleich zu Notate ML Ranking im Ländervergleich
Vergleichen Sie Neural Object Detector den Ranking-Trend der letzten 28 Tage mit Notate ML
Alle Kategorien
Keine Daten verfügbar
Entwickler-Tools
Stellen Sie mit unserer kostenlosen Testversion Vergleiche mit jeder Website an
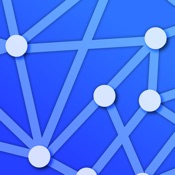
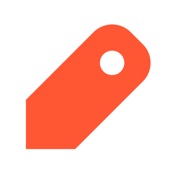
Dezember 12, 2024